Creating representations of words is to capture their meaning, semantic relationship, and context of different words; here, different word embedding techniques play a role. A word embedding is an approach used to provide dense vector representation of words that capture some context words about their own. These are improved versions of simple bag-of-words models like word counts and frequency counters, mostly representing sparse vectors.
Word embeddings use an algorithm to train fixed-length dense vectors and continuous-valued vectors based on a large text corpus. Each word represents a point in vector space, and these points are learned and moved around the target word by preserving semantic relationships. The vector space representation of words provides a projection where words with similar meanings are clustered within the space.
You are viewing: How To Do Embedding In Glove
The use of embeddings over the other text representation techniques like one-hot encodes, TF-IDF, Bag-of-Words is one of the key methods which has led to many outstanding performances on deep neural networks with problems like neural machine translations. Moreover, some word embedding algorithms like GloVe and word2vec are likely to produce a state of performance achieved by neural networks.
Today in this article, we will look at the GloVe word embedding model given by Stanford University. We will load pre-trained models, find similar words by the given word, and try to implement mathematical analogies with words and visualize the vectors.
Implementing GloVe
GloVe stands for Global Vectors for word representation. It is an unsupervised learning algorithm developed by researchers at Stanford University aiming to generate word embeddings by aggregating global word co-occurrence matrices from a given corpus.
The basic idea behind the GloVe word embedding is to derive the relationship between the words from statistics. Unlike the occurrence matrix, the co-occurrence matrix tells you how often a particular word pair occurs together. Each value in the co-occurrence matrix represents a pair of words occurring together.
Let’s see how to interpret the co-occurrence matrix,
Consider the below matrix for example;
The matrix formed by considering the unique words from two corpus as written in block 1 and block 2. Move vertically from word cat; in block 1, there is no repetition of word cat and similarly in block 2. Moving to the next pair cat-fast, it has occurred in both blocks once together and the pair has occurred twice in the given corpus. If you take one more pair, cat-the, how many times ‘the’ has come with cat, that is 3 times. Similarly, the whole matrix is formed.
Now when you compute the ratio of probabilities between two pairs of words say you have probabilities of (cat/fast) = 1 and (cat/the)=0.5; when you compute the ratio of these, the result is 2 it says ‘fast’ is more relevant than ‘the’
This is the idea behind the GloVe pre-trained word embeddings, and it is expressed as;
Read more : How To Clean Spots From Leather Gloves
To start with GloVe, first we have to download the pre-trained model hosted at https://nlp.stanford.edu/projects/glove/; there are a total four pre-trained models available; you can choose anyone you want. If the files are not getting downloaded, try to open the download link in Incognito Tab.
Here we have chosen to download the Wikipedia 2014 model, which has size around 800MB. Let’s start with the codes.
Import all dependencies:
import os import urllib.request import matplotlib.pyplot as plt from scipy import spatial from sklearn.manifold import TSNE import numpy as np
In Colab or Jupyter notebook, we can directly access the files from the URL as below with urllib.
urllib.request.urlretrieve(‘https://nlp.stanford.edu/data/glove.6B.zip’,’g love.6B.zip’)
As it is a zip file, we can simply unzip it by mentioning the source of file and destination for unzipped file in the below command;
!unzip “/content/glove.6B.zip” -d “/content/”
There are five text files representing the word and its corresponding vectors in various dimensions like 50 dimensions, 100 dimensions etc. We are using glove.6B.200d.txt here, 200d refers to 200 dimensions for each word.
Lates take a look at one line of the file and its dimensions;
after 0.38315 -0.3561 -0.1283 -0.19527 0.047629…
Now to create the word embeddings; first, we need to create a dictionary holding each word and its respective vector; this can be simply achieved by looping through the file, which will extract word and vectors as below;
Read more : How Have Boxing Gloves Changed
emmbed_dict = {} with open(‘/content/glove.6B.200d.txt’,’r’) as f: for line in f: values = line.split() word = values[0] vector = np.asarray(values[1:],’float32′) emmbed_dict[word]=vector
Sample of dictionary looks like below;
Now let’s find a similar word by querying; in this method, we will be using euclidean distance to measure how far apart the two words are. Using this euclidean distance as key in the sorted function and keys of emmbed_dict together, we can obtain similar words as below;
def find_similar_word(emmbedes): nearest = sorted(emmbed_dict.keys(), key=lambda word: spatial.distance.euclidean(emmbed_dict[word], emmbedes)) return nearest
Now let’s find the top 10 similar words for the word ‘river’;
find_similar_word(emmbed_dict[‘river’])[0:10]
We are not limited to just getting similar words, we can also try to search words by combining two or more words;
find_similar_word(emmbed_dict[‘king’] + emmbed_dict[‘queen’] + emmbed_dict[‘prince’])[0:10]
To visualize the vectors, we are using a method called distributed stochastic gradient neighbor embeddings in short known as TSNE, which is used to reduce data dimensions. Here we are dealing with 200-dimensional data TSNE will break it down into components as we want here; we will break it into two dimensions.
After training and fitting the TSNE model, it is all about plotting the vector; we are using a scatter plot as the vector is distributed over the space. The matplotlib library can do this. Annotating each point will give a more insightful vector representation.
distri = TSNE(n_components=2) words = list(emmbed_dict.keys()) vectors = [emmbed_dict[word] for word in words] y = distri.fit_transform(vectors[700:850]) plt.figure(figsize=(14,8)) plt.scatter(y[:, 0],y[:,1]) for label,x,y in zip(words,y[:, 0],y[:,1]): plt.annotate(label,xy=(x,y),xytext=(0,0),textcoords=’offset points’) plt.show()
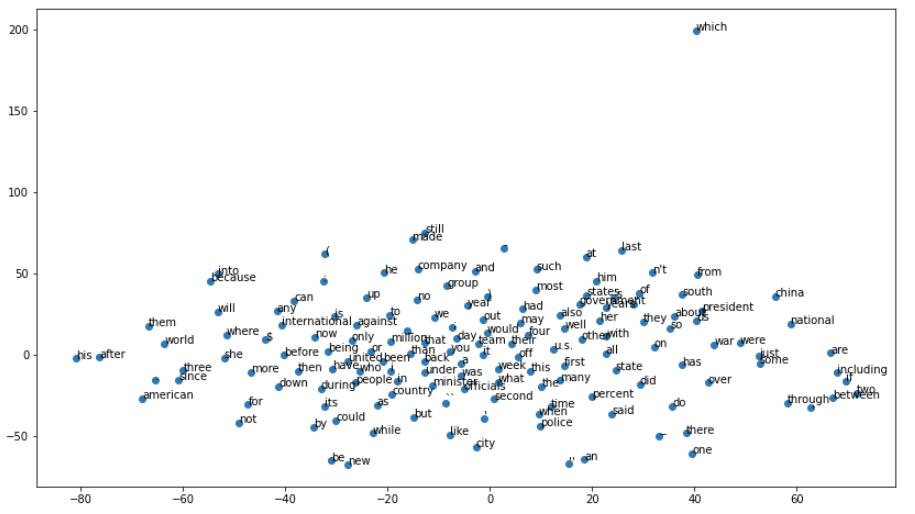
Conclusion:
From this article, we have seen how vector representation techniques such as GloVe can be used to represent a given corpus with semantic meaning. Additionally, we have seen the main working idea behind the GloVe, which is a co-occurrence matrix, and how GloVe considers the particular word over others based on probabilities.
References:
- Link for above codes
- GloVe from Stanford
Source: https://t-tees.com
Category: HOW