Hm, seeing the visual illustrating this post I had some objections: is it really reasonable to represent the measures of a supply chain in the 21st century as following a normal law?
No, certainly not – the measures do not follow the normal law, while we live more than ever in a new (ab)normality. However, one thing remains true, whatever the statistical distributions: to improve our performance, there is a reservoir of information in the extremes of the distribution – when our stocks are too high or too low, or our flows ahead or behind.
You are viewing: What Is Ddom
In the Demand Driven Leader training, we see that three types of metrics are used to judge the effectiveness of a Demand Driven operating model: reliability, stability and speed.
All this is good, but it remains a bit theoretical…
In practice, how do we go about it?
Have a Model and Capture the Relevant Data
First, in order to be able to measure too high / too low / advance / delay – and compare the reliability, stability, and speed of our model against the nominal, there are some prerequisites.
The first prerequisite is… to have designed and sized our model, so that we have a benchmark to compare ourselves to. If you do not know what the nominal operating range is for your inventory, for your queues, for your lead times, how can you measure the performance of your supply chain?
The second prerequisite is to have set up measurement points, and to have organized the capture of relevant information on these points. These measurement points are the buffers – of stock, time and capacity – and the control points.
Read more : What Year Chevy Traverse To Avoid
On a stock buffer we measure the high and low points. On a time buffer, we measure advances and delays. On a capacity buffer we measure the punctual overloads. On the control points we measure the speed and the respect of the programs.
The information must be captured at the right time scale: typically, by the day for stock and capacity buffers, but by the minute or by the hour for time buffers and factory control points.
This allows for the accumulation of data. Lots of data. If you have a lot of SKUs and complex chains, a lot of data that is easy to get lost in.
The challenge is to extract from this mass of data the few useful pieces of information that will allow your teams to progress, and to orchestrate their review in a way that turns information into action.
To facilitate this, business intelligence processes have become commonplace in companies – but care must be taken in designing the right materials for your teams.
Past, Present, Future
The first of our recommendations is to distinguish 3 types of measures:
- Measures over a past period – for example, the last three months. These metrics will allow you to track the performance, by family or by item, of your model, and derive opportunities for improvement.
- The picture as of now: where are we today and what are our priorities for action?
- Projection into the future: how are our inventories, lead times and load-capacity likely to evolve according to demand scenarios?
Let us look at an example of past performance: an aggregate dashboard, in this case at the plant level, which includes several synthetic visuals in monthly buckets. It allows a management team to judge the overall progress of performance, and to identify drifts. At first glance, we can see what is happening: within a few months, we have lowered inventories, reduced out-of-stocks and we have fewer items with too high or too low inventory.
Planners will have access to reports detailing the pareto of items too high / too low and the recommendations for improvement generated by the system.
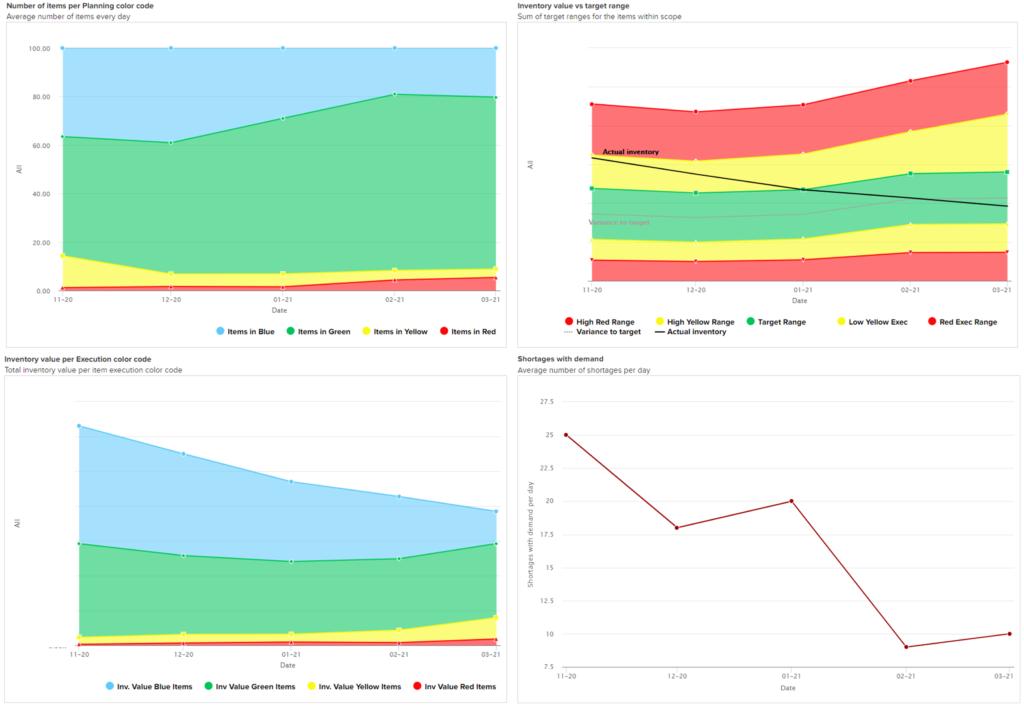
Read more : What Font Does Vogue Use
In the present, you will monitor the health of your model, the respect of recommendations, the management of execution alerts, the status of queues, the target vs actual stocks, the integrity of your data master…
On the other hand, the report below focuses on the projection into the future, in support of S&OP. For the product flow concerned, the stock is expected to drop sharply after the upcoming summer period, and we identify by exception, based on the demand scenario tested, about 70 items whose stock would be in a risky zone for a few weeks. The planner in charge will evaluate options to reduce this risk.
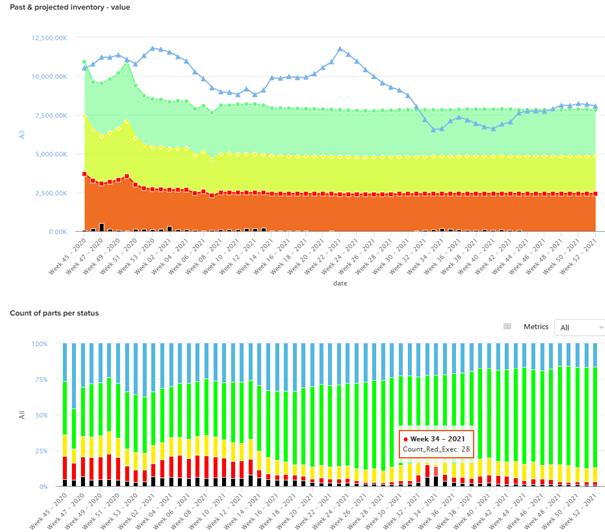
We will come back in a future post on other examples of analysis to identify opportunities for improvement.
Metrics, Audiences, and Rituals
The second of our recommendations is to orchestrate regular review and decision rituals adapted to the teams and individuals in charge. Implementing these rituals is essential to ensure change management. If your Demand Driven transformation is not accompanied by a change in your existing metrics, you will not refocus your teams on adaptation. Eliminate your measures of forecast accuracy, OEE on non-constrained work centers, and fixed cost absorption, so your teams can focus only on what matters!
The orchestration of these rituals also makes it possible to get clear accountability, and thus to move easily from measurement to action.
In our experience, it is the continuous improvement process driven by the Demand Driven model that leads to the greatest improvements: be determined to get results, and give your teams the visibility they need to get there!
Our Advanced Analytics module makes it easy to measure performance and translate it into action. The performance of all buffers and control points is recorded over time and can be analyzed through pre-built reports and an integrated report editor to allow you to customize your metrics. If you prefer to integrate Demand Driven metrics with your existing BI platform, it is also easy: our solutions integrate with any platform on the market via the OData 2.0 standard.
The technique is available, the model is mature and proves its efficiency project after project, do not hesitate any longer!
Source: https://t-tees.com
Category: WHAT